Documents
Presentation Slides
ACTIVE EXPLAINABLE RECOMMENDATION WITH LIMITED LABELING BUDGETS
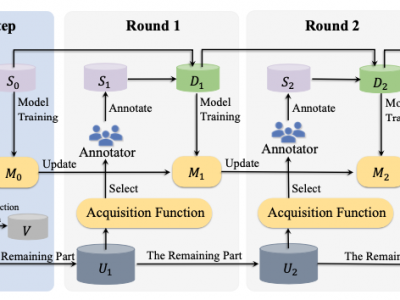
- Citation Author(s):
- Submitted by:
- Jingsen Zhang
- Last updated:
- 29 March 2024 - 6:21am
- Document Type:
- Presentation Slides
- Document Year:
- 2024
- Event:
- Presenters:
- Jingsen Zhang
- Paper Code:
- MLSP-L17.4
- Categories:
- Log in to post comments
Explainable recommendation has gained significant attention due to its potential to enhance user trust and system transparency. Previous studies primarily focus on refining model architectures to generate more informative explanations, assuming that the explanation data is sufficient and easy to acquire. However, in practice, obtaining the ground truth for explanations can be costly since individuals may not be inclined to put additional efforts to provide behavior explanations.
In this paper, we study a novel problem in the field of explainable recommendation, that is, “given a limited budget to incentivize users to provide behavior explanations, how to effectively collect data such that the downstream models can be better optimized? ” To solve this problem, we propose an active learning framework for recommender system, which consists of an acquisition function for sample collection and an explainable recommendation model to provide the final results. We consider both uncertainty and influence based strategies to design the acquisition function, which can determine the sample effectiveness from complementary perspectives. To demonstrate the effectiveness of our framework, we conduct extensive experiments based on real-world datasets.