Documents
Poster
Adversarial Continual Learning to Transfer Self-Supervised Speech Representations for Voice Pathology Detection
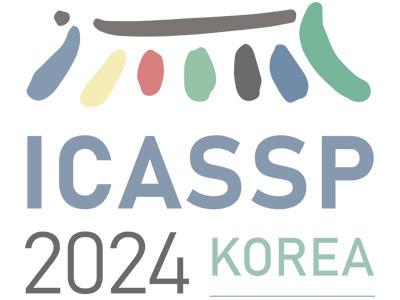
- Citation Author(s):
- Submitted by:
- DongKeon Park
- Last updated:
- 10 April 2024 - 10:23pm
- Document Type:
- Poster
- Document Year:
- 2023
- Event:
- Presenters:
- Dongkeon Park
- Paper Code:
- AASP-P15.7
- Categories:
- Log in to post comments
In recent years, voice pathology detection (VPD) has received considerable attention because of the increasing risk of voice problems. Several methods, such as support vector machine and convolutional neural network-based models, achieve good VPD performance. To further improve the performance, we use a self-supervised pretrained model as feature representation instead of explicit speech features. When the pretrained model is fine-tuned for VPD, an overfitting problem occurs due to a domain shift from conversation speech to the VPD task. To mitigate this problem, we propose an adversarial task adaptive pretraining (A-TAPT) approach by incorporating adversarial regularization during the continual learning process. Experiments on VPD using the Saarbrucken Voice Database show that the proposed A-TAPT improves the unweighted average recall (UAR) by an absolute increase of 12.36% and 15.38% compared with SVM and ResNet50, respectively. It is also shown that the proposed A-TAPT achieves a UAR that is 2.77% higher than that of conventional TAPT learning.