Documents
Presentation Slides
Augmentation Data Synthesis via GANs: Boosting Latent Fingerprint Reconstruction
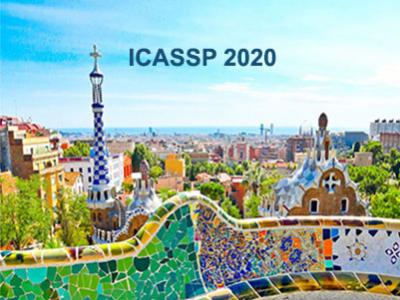
- Citation Author(s):
- Submitted by:
- Ying Xu
- Last updated:
- 15 May 2020 - 1:16am
- Document Type:
- Presentation Slides
- Document Year:
- 2020
- Event:
- Presenters:
- Ying Xu
- Paper Code:
- 1263
- Categories:
- Log in to post comments
Latent fingerprint reconstruction is a vital preprocessing step for its identification. This task is very challenging due to not only existing complicated degradation patterns but also its scarcity of paired training data. To address these challenges, we propose a novel generative adversarial network (GAN) based data augmentation scheme to improve such reconstruction. It translates the abundant clean fingerprints to their corresponding latent ones, only exploiting a small-scale latent dataset and an unpaired large-scale clean dataset, from which a large-scale paired clean-latent augmentation set is built for the reconstruction task. Specifically, our method models the distribution of the latent degradation patterns into a Gaussian one and generates latent fingerprints based on the sampled degradation patterns and clean fingerprints. Besides, we develop an auxiliary training procedure to stabilize training and further disentangle ridge structures and degradation patterns by regressing a latent fingerprint from its latent representation and its corresponding binarized fingerprint. Boosted by the proposed data augmentation, our reconstruction shows significant improvements in the visual evaluation and fingerprint identification performance.
ICASSP1263.pdf
