Documents
Poster
BirdVox-full-night: a dataset and website for avian flight call detection.
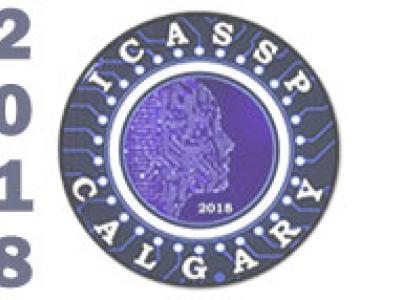
- Citation Author(s):
- Submitted by:
- Vincent Lostanlen
- Last updated:
- 17 April 2018 - 3:54pm
- Document Type:
- Poster
- Document Year:
- 2018
- Event:
- Presenters:
- Vincent Lostanlen
- Paper Code:
- 4130
- Categories:
- Log in to post comments
This article addresses the automatic detection of vocal, nocturnally migrating birds from a network of acoustic sensors.
Thus far, owing to the lack of annotated continuous recordings, existing methods had been benchmarked in a binary classification setting (presence vs. absence).
Instead, with the aim of comparing them in event detection, we release BirdVox-full-night, a dataset of 62 hours of audio comprising 35402 flight calls of nocturnally migrating birds, as recorded from 6 sensors.
We find a large performance gap between energy-based detection functions and data-driven machine listening.
The best model is a deep convolutional neural network trained with data augmentation.
We correlate recall with the density of flight calls over time and frequency and identify the main causes of false alarm.