Documents
Poster
BOOSTING ZERO-SHOT HUMAN-OBJECT INTERACTION DETECTION WITH VISION-LANGUAGE TRANSFER
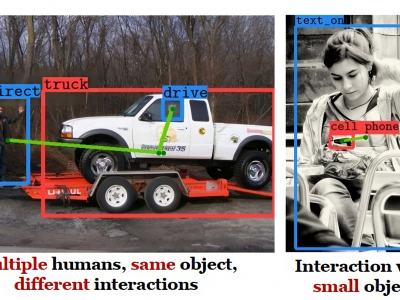
- Citation Author(s):
- Submitted by:
- Sandipan Sarma
- Last updated:
- 7 April 2024 - 11:29am
- Document Type:
- Poster
- Document Year:
- 2024
- Event:
- Presenters:
- Sandipan Sarma
- Paper Code:
- MLSP-P28.12
- Categories:
- Log in to post comments
Human-Object Interaction (HOI) detection is a crucial task that involves localizing interactive human-object pairs and identifying the actions being performed. Most existing HOI detectors are supervised in nature and lack the ability of zero-shot discovery of unseen interactions. Recently, transformer-based methods have superseded the traditional CNN detectors by aggregating image-wide context but still suffer from the long-tail distribution problem in HOI. In this work, our primary focus is improving HOI detection in images, particularly in zero-shot scenarios. We use an end-to-end transformer-based object detector to localize
human-object pairs and yield visual features of actions and objects. Moreover, we adopt the text encoder from a popular visual-language model called CLIP with a novel prompting mechanism to extract semantic information for unseen actions and objects. Finally, we learn a strong visual-semantic alignment and achieve state-of-the-art performance on the challenging HICO-DET dataset across five zero-shot settings, with up to 70.88% relative gains. Code is available at
https://github.com/sandipan211/ZSHOI-VLT.