Documents
Poster
Constrained Autoencoders: Incorporating equality constraints in learned scientific data compression
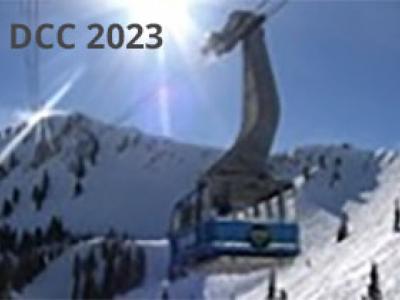
- Citation Author(s):
- Submitted by:
- Jaemoon Lee
- Last updated:
- 16 March 2023 - 6:39pm
- Document Type:
- Poster
- Document Year:
- 2023
- Event:
- Presenters:
- Jaemoon Lee
- Paper Code:
- 248
- Categories:
- Keywords:
- Log in to post comments
As today's scientific simulations with high-performance computing produce an extremely large amount of data, reliable data compression techniques are becoming important. In scientific applications, downstream quantities derived from the original or primary data (PD) are crucial for post-analysis, and therefore these Quantities of Interest (QoI) are required to be preserved during compression. While autoencoders (AEs) have recently been used for the compression of scientific datasets, there is a clear lack in the literature w.r.t. the imposition of scientific data constraints during learned data compression. We address this lack by developing a new Lagrangian formulation which results in the minimization of a joint objective function on both AE weights and Lagrange parameters (one set per training instance). Despite the apparent asymmetry between global AE weights and local (instance-dependent) Lagrange parameters, we show that the new loss function treats both the AE loss and the constraints in an integrated manner---leading to a very simple algorithm that alternates between solving for the Lagrange parameters for each batch and updating the weights. We demonstrate the constrained AE (CAE) on XGC---a tokamak simulation with domain-specific constraints. Experiments show that the CAE can preserve the QoI in XGC within machine precision.