Documents
Poster
CONTEXTUAL BIASING OF NAMED-ENTITIES WITH LARGE LANGUAGE MODELS
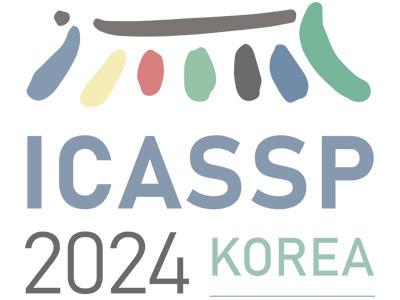
- Citation Author(s):
- Submitted by:
- Chuanneng Sun
- Last updated:
- 4 April 2024 - 9:04pm
- Document Type:
- Poster
- Document Year:
- 2024
- Event:
- Presenters:
- Chuanneng Sun
- Paper Code:
- 2008
- Categories:
- Log in to post comments
We explore contextual biasing with Large Language Models (LLMs) to enhance Automatic Speech Recognition (ASR) in second-pass rescoring. Our approach introduces the utilization of prompts for LLMs during rescoring without the need for fine-tuning. These prompts incorporate a biasing list and a set of few-shot examples, serving as supplementary sources of information when evaluating the hypothesis score. Furthermore, we introduce multi-task training for LLMs to predict entity class and the subsequent token. To address sequence length constraints and improve the efficiency of contextual biasing, we propose dynamic prompting based on class tag predictions. Through dynamic prompting, we leverage the class tag predictions to identify the most probable entity class and subsequently utilize entities within this class as biasing context for the next token prediction. We evaluate the performance of proposed methods in terms of Word Error Rate (WER) on an internal entity-heavy and the SLUE-Voxpopuli datasets. Our results show significant improvements: biasing lists and few-shot examples achieved a relative improvement of 17.8% and 9.6%, while multi-task training and dynamic prompting achieved 20.0% and 11.3% relative WER improvement, respectively.