Documents
Poster
Crime event embedding with unsupervised feature selection
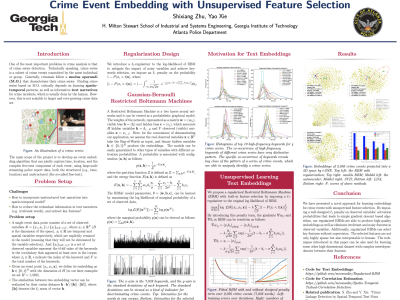
- Citation Author(s):
- Submitted by:
- Shixiang Zhu
- Last updated:
- 14 April 2019 - 4:12pm
- Document Type:
- Poster
- Document Year:
- 2019
- Event:
- Presenters:
- Yao Xie
- Paper Code:
- 3112
- Categories:
- Log in to post comments
We present a novel event embedding algorithm for crime data that can jointly capture time, location, and the complex free-text component of each event. The embedding is achieved by regularized Restricted Boltzmann Machines (RBMs), and we introduce a new way to regularize by imposing a ℓ1 penalty on the conditional distributions of the observed variables of RBMs. This choice of regularization performs feature selection and it also leads to efficient computation since the gradient can be computed in a closed form. The feature selection forces embedding to be based on the most important keywords, which captures the common modus operandi (M. O.) in crime series. Using numerical experiments on a large-scale crime dataset, we show that our regularized RBMs can achieve better event embedding and the selected features are highly interpretable from human understanding.