Documents
Presentation Slides
Deep Unfolding for Multichannel Source Separation
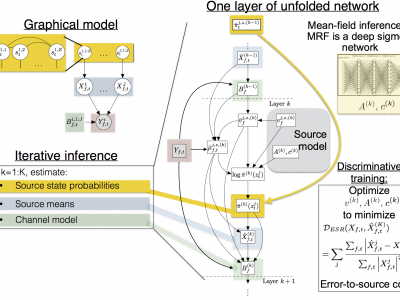
- Citation Author(s):
- Submitted by:
- Scott Wisdom
- Last updated:
- 24 March 2016 - 9:11pm
- Document Type:
- Presentation Slides
- Document Year:
- 2016
- Event:
- Presenters:
- Scott Wisdom
- Paper Code:
- AASP-L5.01
- Categories:
- Log in to post comments
Deep unfolding has recently been proposed to derive novel deep network architectures from model-based approaches. In this paper, we consider its application to multichannel source separation. We unfold a multichannel Gaussian mixture model (MCGMM), resulting in a deep MCGMM computational network that directly processes complex-valued frequency-domain multichannel audio and has an architecture defined explicitly by a generative model, thus combining the advantages of deep networks and model-based approaches. We further extend the deep MCGMM by modeling the GMM states using an MRF, whose unfolded mean-field inference updates add dynamics across layers. Experiments on source separation for multichannel mixtures of two simultaneous speakers shows that the deep MCGMM leads to improved performance with respect to the original MCGMM model.