Documents
Presentation Slides
On-Device Constrained Self-Supervised Learning for Keyword Spotting via Quantization Aware Pre-Training and Fine-tuning
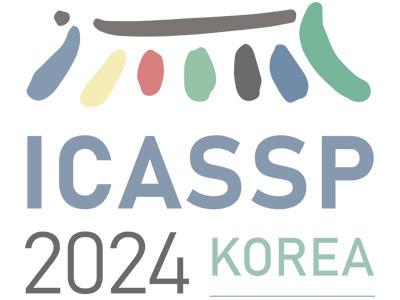
- DOI:
- 10.60864/p51r-3189
- Citation Author(s):
- Submitted by:
- Gene-Ping Yang
- Last updated:
- 16 April 2024 - 9:53pm
- Document Type:
- Presentation Slides
- Document Year:
- 2024
- Event:
- Presenters:
- Gene-Ping Yang
- Paper Code:
- SLP-L16.2
- Categories:
- Log in to post comments
Large self-supervised models have excelled in various speech processing tasks, but their deployment on resource-limited devices is often impractical due to their substantial memory footprint. Previous studies have demonstrated the effectiveness of self-supervised pre-training for keyword spotting, even with constrained model capacity. In our pursuit of maintaining high performance while minimizing the model's resource demands, we investigate the implementation of Quantization Aware Training for both self-supervised pre-training and fine-tuning, specifically tailored to fit within the constraints of on-device model budget. Our experiments emphasize the critical role of selecting and synchronizing QAT methods throughout both stages of model training and tuning. We evaluate our methodology on a 16.6k-hour in-house keyword spotting dataset, and show that there is no decline in performance, even when the bit size of model weights and activations is cut by a factor of four.
final_v5.pdf
