Documents
Presentation Slides
G-SharP: Globally Shared Kernel with Pruning for Efficient CNNs
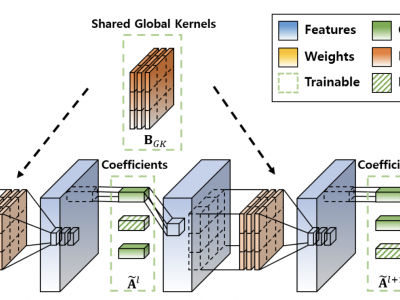
- DOI:
- 10.60864/3wrk-kg32
- Citation Author(s):
- Submitted by:
- Eunseop Shin
- Last updated:
- 15 April 2024 - 11:46am
- Document Type:
- Presentation Slides
- Document Year:
- 2024
- Event:
- Presenters:
- Eunseop Shin
- Paper Code:
- MLSP-L1.5
- Categories:
- Log in to post comments
Filter Decomposition (FD) methods have gained traction in compressing large neural networks by dividing weights into basis and coefficients. Recent advancements have focused on reducing weight redundancy by sharing either basis or coefficients stage-wise. However, traditional sharing approaches have overlooked the potential of sharing basis on a network-wide scale. In this study, we introduce an FD technique called G-SharP that elevates performance by using globally shared kernels throughout the network. To bolster the efficacy of G-SharP, we unveil a novel batch normalization-based coefficient pruning strategy aiming to boost computational efficiency. Comprehensive evaluations show that our method notably diminishes computational demands and model size while incurring only a slight decline in performance. On benchmarks like CIFAR-10, ImageNet, PASCAL-VOC, and MS-COCO, G-SharP achieves significant reductions in model dimensions and FLOPs yet maintains accuracy levels akin to the original uncompressed models. Notably, G-SharP surpasses numerous leading lightweight models, striking a commendable balance between precision and efficiency.