Documents
Poster
ICASSP 2019 Poster for Paper #3198: PRIVACY-AWARE FEATURE EXTRACTION FOR GENDER DISCRIMINATION VERSUS SPEAKER IDENTIFICATION
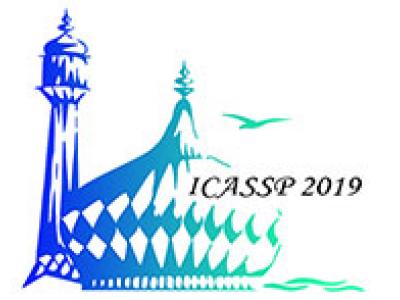
- Citation Author(s):
- Submitted by:
- Alexandru Nelus
- Last updated:
- 8 May 2019 - 2:50am
- Document Type:
- Poster
- Document Year:
- 2019
- Event:
- Presenters:
- Alexandru Nelus
- Paper Code:
- 3198
- Categories:
- Log in to post comments
This paper introduces a deep neural network based feature extraction scheme that aims to improve the trade-off between utility and privacy in speaker classification tasks. In the proposed scenario we develop a feature representation that helps to maximize the performance of a gender classifier while minimizing additional speaker
identity information. Our approach is to use variational information feature extraction that allows for gender discrimination (utility) but minimizes the information level of the features, thus discouraging speaker identification adversarial attacks (privacy). We analyze the model’s loss function and the budget scaling factor used to control the balance of utility vs. privacy. It is experimentally shown that the proposed method reduces privacy risks without significantly deprecating utility and that it also generalizes well to new speaker contexts