Documents
Presentation Slides
InfoShape: Task-Based Neural Data Shaping via Mutual Information
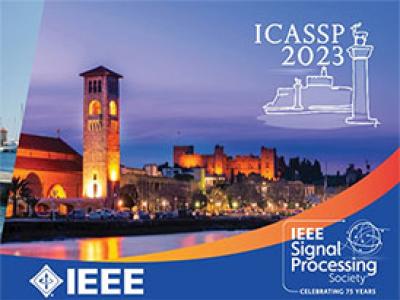
- Citation Author(s):
- Submitted by:
- Homa Esfahanizadeh
- Last updated:
- 19 May 2023 - 12:25pm
- Document Type:
- Presentation Slides
- Document Year:
- 2023
- Event:
- Presenters:
- Homa Esfahanizadeh
- Paper Code:
- MLSP-L9.2
- Categories:
- Log in to post comments
The use of mutual information as a tool in private data sharing has remained an open challenge due to the difficulty of its estimation in practice. In this paper, we propose InfoShape, a task-based encoder that aims to remove unnecessary sensitive information from training data while maintaining enough relevant information for a particular ML training task. We achieve this goal by utilizing mutual information estimators that are based on neural networks, in order to measure two performance metrics, privacy and utility. Using these together in a Lagrangian optimization, we train a separate neural network as a lossy encoder. We empirically show that InfoShape is capable of shaping the encoded samples to be informative for a specific downstream task while eliminating unnecessary sensitive information. Moreover, we demonstrate that the classification accuracy of downstream models has a meaningful connection with our utility and privacy measures.