Documents
Poster
Learning to Fool the Speaker Recognition (poster)
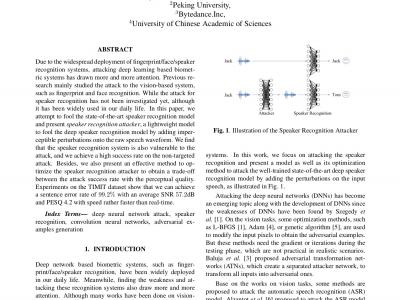
- Citation Author(s):
- Submitted by:
- Jiguo Li
- Last updated:
- 9 June 2020 - 11:26am
- Document Type:
- Poster
- Document Year:
- 2020
- Event:
- Presenters:
- Jiguo Li
- Categories:
- Log in to post comments
Due to the widespread deployment of fingerprint/face/speaker recognition systems, attacking deep learning based biometric systems has drawn more and more attention. Previous research mainly studied the attack to the vision-based system, such as fingerprint and face recognition. While the attack for speaker recognition has not been investigated yet, although it has been widely used in our daily life. In this paper, we attempt to fool the state-of-the-art speaker recognition model and present speaker recognition attacker, a lightweight model to fool the deep speaker recognition model by adding imperceptible perturbations onto the raw speech waveform. We find that the speaker recognition system is also vulnerable to the attack, and we achieve a high success rate on the non-targeted attack. Besides, we also present an effective method to optimize the speaker recognition attacker to obtain a trade-off between the attack success rate with the perceptual quality. Experiments on the TIMIT dataset show that we can achieve a sentence error rate of 99.2% with an average SNR 57.2dB and PESQ 4.2 with speed rather 20 times than the real-time.