Documents
Poster
Less peaky and more accurate CTC forced alignment by label priors
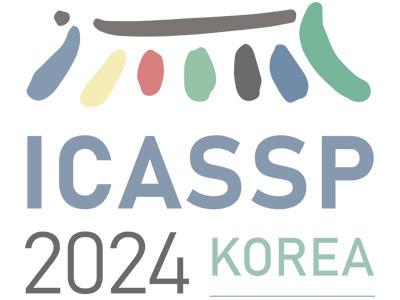
- Citation Author(s):
- Submitted by:
- Ruizhe Huang
- Last updated:
- 12 April 2024 - 10:51pm
- Document Type:
- Poster
- Document Year:
- 2024
- Event:
- Presenters:
- Ruizhe Huang
- Categories:
- Log in to post comments
Connectionist temporal classification (CTC) models are known to have peaky output distributions. Such behavior is not a problem for automatic speech recognition (ASR), but it can cause inaccurate forced alignments (FA), especially at finer granularity, e.g., phoneme level. This paper aims at alleviating the peaky behavior for CTC and improve its suitability for forced alignment generation, by leveraging label priors, so that the scores of alignment paths containing fewer blanks are boosted and maximized during training. As a result, our CTC model produces less peaky posteriors and is able to more accurately predict the offset of the tokens besides their onset. It outperforms the standard CTC model and a heuristics-based approach for obtaining CTC’s token offset timestamps by 12 − 40% in phoneme and word boundary errors (PBE and WBE) measured on the Buckeye and TIMIT data. Compared with the most widely used FA toolkit Montreal Forced Aligner (MFA), our method performs similarly on PBE/WBE on Buckeye, yet falls behind MFA on TIMIT. Nevertheless, our method has a much simpler training pipeline and better runtime efficiency. Our training recipe and pretrained model are released in TorchAudio.
aligner.pdf

Comments
This is the poster for our
This is the poster for our ICASSP paper.