Documents
Presentation Slides
M3SUM: A Novel Unsupervised Language-guided Video Summarization
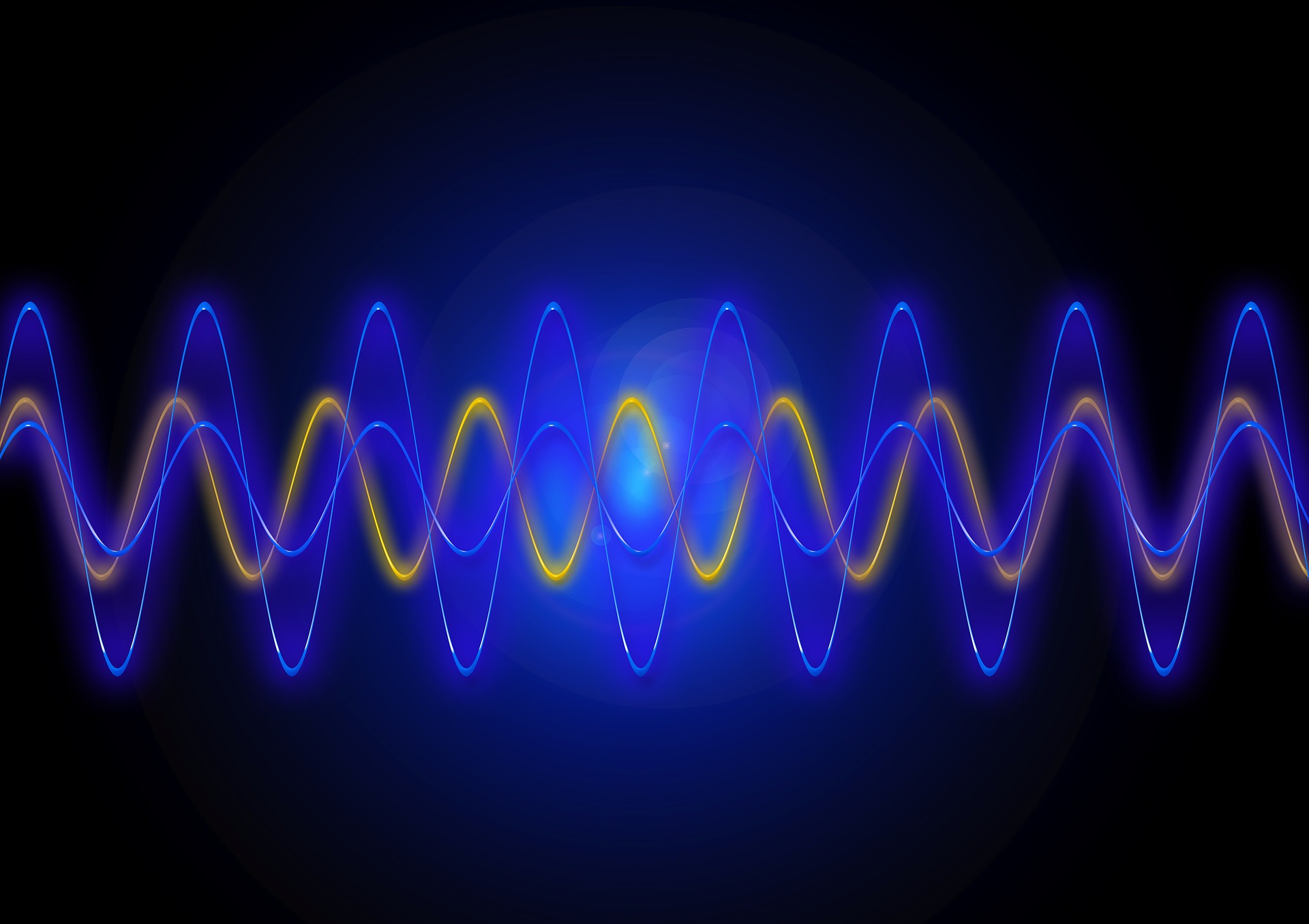
- Citation Author(s):
- Submitted by:
- Hongru Wang
- Last updated:
- 28 March 2024 - 11:24pm
- Document Type:
- Presentation Slides
- Categories:
- Log in to post comments
Language-guided video summarization empowers users to use natural language queries to effortlessly summarize lengthy videos into concise and relevant summaries that cater specifically to their information needs, which is more friendly to access and digest. However, most of the previous works rely on tremendous (also expensive) annotated videos and complex designs to align different modals at the feature level. In this paper, we first explore the combination of off-the-shelf models for each modal to solve the complex multi-modal problem by proposing a novel unsupervised language-guided video summarization method: Modular Multi-Modal Summarization (M3Sum), which does not require any training data or parameter updates. Specifically, instead of training an alignment module at the feature level, we convert all modal information (e.g. audio and frames) into textual descriptions and design a parameter-free alignment mechanism to fuse text descriptions from different modals. Benefiting from the remarkable long-context understanding capability of large language models (LLMs), our approach demonstrates comparable performance to most unsupervised methods and even outperforms certain supervised methods.