Documents
Poster
MODEL-BASED LABEL-TO-IMAGE DIFFUSION FOR SEMI-SUPERVISED CHOROIDAL VESSEL SEGMENTATION
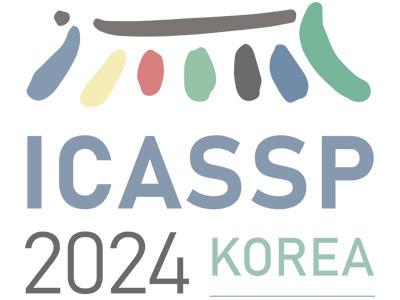
- Citation Author(s):
- Submitted by:
- kun huang
- Last updated:
- 10 April 2024 - 7:07am
- Document Type:
- Poster
- Document Year:
- 2024
- Event:
- Presenters:
- Kun Huang
- Paper Code:
- BISP-P8.10
- Categories:
- Log in to post comments
Current successful choroidal vessel segmentation methods rely on large amounts of voxel-level annotations on the 3D optical coherence tomography images, which are hard and time-consuming. Semi-supervised learning solves this issue by enabling model learning from both unlabeled data and a limited amount of labeled data. A challenge is the defective pseudo labels generated for the unlabeled data. In this work, we propose a model-based label-to-image diffusion (MLD) framework for semi-supervised choroidal vessel segmentation. We first generate pseudo labels from unlabeled images with a coarse correspondence using a model-based strategy. Then, we generate precisely corresponding images of pseudo labels by a hierarchical diffusion probabilistic model. We evaluated our method on myopia data with a new topological connectivity metric. The quantitative and qualitative experimental results indicate the effectiveness of the label-to-image diffusion framework and its benefit for enhancing the existing supervised choroidal segmentation methods. The code is available at: https://github.com/nicetomeetu21/MLD.
ICASSP_poster.pdf
