Documents
Poster
MoGA: Searching Beyond MobileNetV3
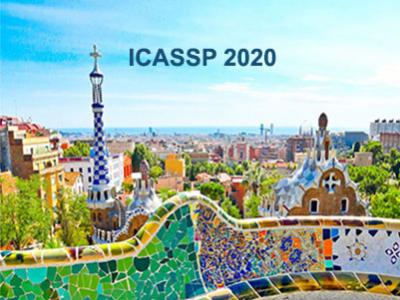
- Citation Author(s):
- Submitted by:
- Bo Zhang
- Last updated:
- 10 February 2020 - 11:33am
- Document Type:
- Poster
- Document Year:
- 2020
- Event:
- Presenters:
- Bo Zhang
- Categories:
- Log in to post comments
In this paper, we aim to bring forward the frontier of mobile neural architecture design by utilizing the latest neural architecture search (NAS) approaches. First, we shift the search trend from mobile CPUs to mobile GPUs, with which we can gauge the speed of a model more accurately and provide a production-ready solution. On this account, our overall search approach is named \alert{Mobile GPU-Aware neural architecture search (MoGA)}.
Second, we replace traditional multi-objective optimization with a weighted fitness strategy where we lay more attention on accuracy and latency, other than the number of parameters.
Third, we benefit from the recent one-shot supernet training and build an accurate latency look-up table. The overall NAS pipeline costs \textbf{12 GPU days}, about \textbf{200$\times$} less than MnasNet. Finally, we present our searched architectures that outperform MobileNetV3. Namely, MoGA-A achieves an outstanding \textbf{75.9\%} top-1 accuracy on ImageNet, MoGA-B 75.5\% and MoGA-C 75.3\%. Remarkably, MoGA-A achieves \textbf{0.9\%} higher accuracy than MobileNetV3 with only 1$ms$ increased latency on mobile GPUs.