Documents
Poster
Multi-Source Domain Adaptation meets Dataset Distillation through Dataset Dictionary Learning
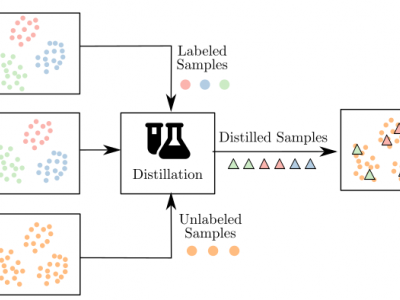
- Citation Author(s):
- Submitted by:
- Eduardo Fernand...
- Last updated:
- 11 April 2024 - 10:08am
- Document Type:
- Poster
- Document Year:
- 2024
- Event:
- Presenters:
- Eduardo Fernandes Montesuma
- Paper Code:
- MLSP-P27.5
- Categories:
- Keywords:
- Log in to post comments
In this paper, we consider the intersection of two problems in machine learning: Multi-Source Domain Adaptation (MSDA) and Dataset Distillation (DD). On the one hand, the first considers adapting multiple heterogeneous labeled source domains to an unlabeled target domain. On the other hand, the second attacks the problem of synthesizing a small summary containing all the information about the datasets. We thus consider a new problem called MSDA-DD. To solve it, we adapt previous works in the MSDA literature, such as Wasserstein Barycenter Transport and Dataset Dictionary Learning, as well as DD method Distribution Matching. We thoroughly experiment with this novel problem on four benchmarks (Caltech-Office 10, Tennessee-Eastman Process, Continuous Stirred Tank Reactor, and Case Western Reserve University), where we show that, even with as little as 1 sample per class, one achieves state-of-the-art adaptation performance.