Documents
Presentation Slides
MULTILINGUAL AUDIO-VISUAL SPEECH RECOGNITION WITH HYBRID CTC/RNN-T FAST CONFORMER
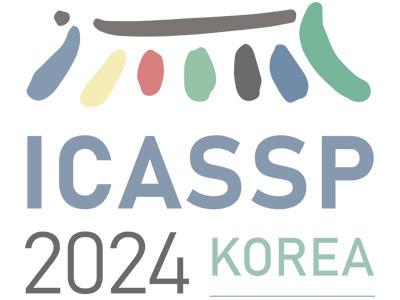
- DOI:
- 10.60864/kkgp-dv12
- Citation Author(s):
- Submitted by:
- Maxime Burchi
- Last updated:
- 18 April 2024 - 8:36am
- Document Type:
- Presentation Slides
- Document Year:
- 2024
- Event:
- Presenters:
- Maxime Burchi
- Paper Code:
- SLP-L25.3
- Categories:
- Log in to post comments
Humans are adept at leveraging visual cues from lip movements for recognizing speech in adverse listening conditions. Audio-Visual Speech Recognition (AVSR) models follow similar approach to achieve robust speech recognition in noisy conditions. In this work, we present a multilingual AVSR model incorporating several enhancements to improve performance and audio noise robustness. Notably, we adapt the recently proposed Fast Conformer model to process both audio and visual modalities using a novel hybrid CTC/RNN-T architecture. We increase the amount of audio-visual training data for six distinct languages, generating automatic transcriptions of unlabelled multilingual datasets (VoxCeleb2 and AVSpeech). Our proposed model achieves new state-of-the-art performance on the LRS3 dataset, reaching WER of 0.8%. On the recently introduced MuAViC benchmark, our model yields an absolute average-WER reduction of 11.9% in comparison to the original baseline. Finally, we demonstrate the ability of the proposed model to perform audio-only, visual-only, and audio-visual speech recognition at test time.