Documents
Poster
Point Cloud Segmentation using Hierarchical Tree for Architectural Models.
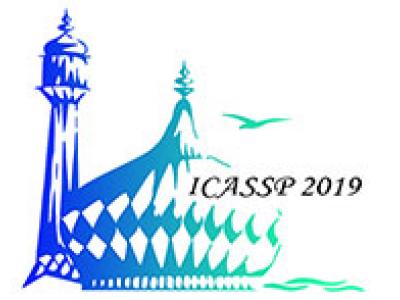
- Citation Author(s):
- Submitted by:
- omair hassaan
- Last updated:
- 8 May 2019 - 2:36pm
- Document Type:
- Poster
- Document Year:
- 2019
- Event:
- Presenters:
- Murtaza Taj
- Paper Code:
- 2747
- Categories:
- Log in to post comments
Over the past few years, gathering massive volume of 3D data has become straightforward due to the proliferation of laser scanners and acquisition devices. Segmentation of such large data into meaningful segments, however, remains a challenge. Raw scans usually have missing data and varying density. In this work, we present a simple yet effective method to semantically decompose and reconstruct 3D models from point clouds. Using a hierarchical tree approach, we segment and reconstruct planar as well as non-planar scenes in an outdoor environment. This tree uses an exclusive energy function and a 3D convolutional neural network, HollowNets, to classify the segments. We test the efficacy of our proposed approach on a variety of complex real and synthetic data samples, obtaining an improvement of 7.9% in mean IOU over the state of the art approaches.