Documents
Presentation Slides
Quantum Federated Learning with Quantum Networks PPT
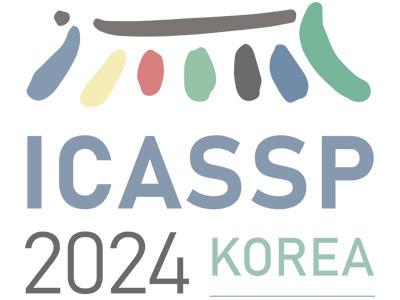
- Citation Author(s):
- Submitted by:
- Tyler Wang
- Last updated:
- 16 April 2024 - 10:56am
- Document Type:
- Presentation Slides
- Document Year:
- 2024
- Event:
- Presenters:
- Tyler Wang
- Paper Code:
- SS-L4.4
- Categories:
- Keywords:
- Log in to post comments
A major concern of deep learning models is the large amount of data that is required to build and train them, much of which is reliant on sensitive and personally identifiable information that is vulnerable to access by third parties. Ideas of using the quantum internet to address this issue have been previously proposed, which would enable fast and completely secure online communications. Previous work has yielded a hybrid quantum-classical transfer learning scheme for classical data and communication with a hub-spoke topology. While quantum communication is secure from eavesdrop attacks and no measurements from quantum to classical translation, due to no cloning theorem, hub-spoke topology is not ideal for quantum communication without quantum memory. Here we seek to improve this model by implementing a decentralized ring topology for the federated learning scheme, where each client is given a portion of the entire dataset and only performs training on that set. We also demonstrate the first successful use of quantum weights for quantum federated learning, which allows us to perform our training entirely in quantum.