Documents
Presentation Slides
RAW WAVEFORM BASED END-TO-END DEEP CONVOLUTIONAL NETWORK FOR SPATIAL LOCALIZATION OF MULTIPLE ACOUSTIC SOURCES
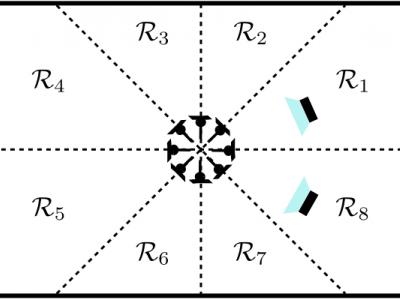
- Citation Author(s):
- Submitted by:
- Harshavardhan Sundar
- Last updated:
- 3 May 2020 - 3:51pm
- Document Type:
- Presentation Slides
- Document Year:
- 2020
- Event:
- Presenters:
- Harshavardhan Sundar
- Paper Code:
- 5054
- Categories:
- Log in to post comments
In this paper, we present an end-to-end deep convolutional neural network operating on multi-channel raw audio data to localize multiple simultaneously active acoustic sources in space. Previously reported end-to-end deep learning based approaches work well in localizing a single source directly from multi-channel raw-audio, but are not easily extendable to localize multiple sources due to the well known permutation problem. Here, we propose a novel encoding scheme to represent the spatial co-ordinates of multiple sources which facilitates 2D localization of multiple sources in an end-to-end fashion by avoiding the permutation problem and achieving arbitrary spatial resolution. Evaluation on a simulated data set and real recordings from the AV16.3 Corpus clearly show that the proposed end-to-end network generalizes well to unseen test conditions and outperforms a recent time difference of arrival (TDOA) based multiple source localization approach reported in the literature.