Documents
Poster
Structural Optimization of Factor Graphs for Symbol Detection via Continuous Clustering and Machine Learning
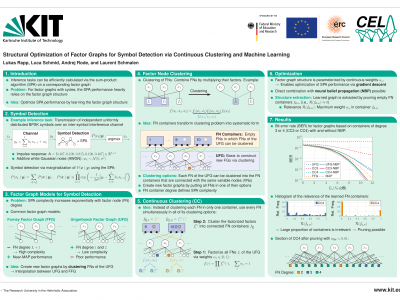
- DOI:
- 10.60864/4h3n-2749
- Citation Author(s):
- Submitted by:
- Lukas Rapp
- Last updated:
- 17 November 2023 - 12:07pm
- Document Type:
- Poster
- Document Year:
- 2023
- Event:
- Presenters:
- Lukas Rapp
- Paper Code:
- SPCN-P2.7
- Categories:
- Log in to post comments
We propose a novel method to optimize the structure of factor graphs for graph-based inference. As an example inference task, we consider symbol detection on linear inter-symbol interference channels. The factor graph framework has the potential to yield low-complexity symbol detectors. However, the sum-product algorithm on cyclic factor graphs is suboptimal and its performance is highly sensitive to the underlying graph. Therefore, we optimize the structure of the underlying factor graphs in an end-to-end manner using machine learning. For that purpose, we transform the structural optimization into a clustering problem of low-degree factor nodes that incorporates the known channel model into the optimization. Furthermore, we study the combination of this approach with neural belief propagation, yielding near-maximum a posteriori symbol detection performance for specific channels.
poster.pdf
