Documents
Poster
TIME SERIES PREDICTION FOR KERNEL-BASED ADAPTIVE FILTERS USING VARIABLE BANDWIDTH, ADAPTIVE LEARNING-RATE, AND DIMENSIONALITY REDUCTION
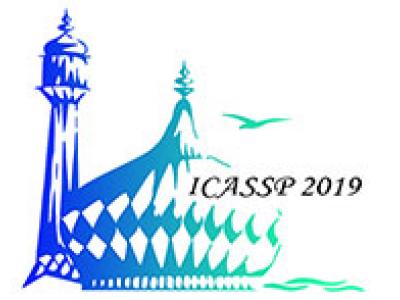
- Citation Author(s):
- Submitted by:
- Sergio Garcia-Vega
- Last updated:
- 8 May 2019 - 3:01pm
- Document Type:
- Poster
- Document Year:
- 2019
- Event:
- Presenters:
- Sergio Garcia-Vega
- Paper Code:
- 3316
- Categories:
- Log in to post comments
Kernel-based adaptive filters are sequential learning algorithms, operating on reproducing kernel Hilbert spaces. Their learning performance is susceptible to the selection of appropriate values for kernel bandwidth and learning-rate parameters. Additionally, as these algorithms train the model using a sequence of input vectors, their computation scales with the number of samples. We propose a framework that addresses the previous open challenges of kernel-based adaptive filters. In contrast to similar methods, our proposal sequentially optimizes the bandwidth and learning-rate parameters using stochastic gradient algorithms that maximize the correntropy function. To remove redundant samples, a sparsification approach based on dimensionality reduction is introduced. The framework is validated on both synthetic and real-world data sets. Results show that our proposal converges to relatively low values of mean-square-error while provides stable solutions in real-world applications.