Documents
Poster
TNFORMER: SINGLE-PASS MULTILINGUAL TEXT NORMALIZATION WITH A TRANSFORMER DECODER MODEL
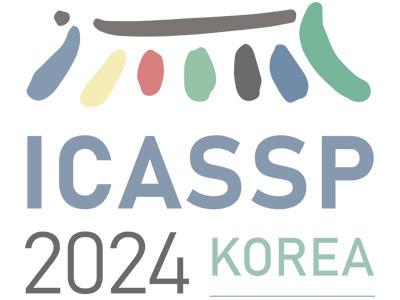
- Citation Author(s):
- Submitted by:
- Jie Wang
- Last updated:
- 6 April 2024 - 9:52pm
- Document Type:
- Poster
- Document Year:
- 2024
- Event:
- Presenters:
- JIE WANG
- Paper Code:
- SLP-P35.1
- Categories:
- Log in to post comments
Text Normalization (TN) is a pivotal pre-processing procedure in speech synthesis systems, which converts diverse forms of text into a canonical form suitable for correct synthesis. This work introduces a novel model, TNFormer, which innovatively transforms the TN task into a next token prediction problem, leveraging the structure of GPT with only Transformer decoders for efficient, single-pass TN. The strength of TNFormer lies not only in its ability to identify Non-Standard Words that require normalization but also in its aptitude for context-driven normalization in a single pass. Though not exclusively designed for multilingual contexts, TNFormer naturally supports different languages and multilingual mixtures, demonstrating impressive performance on English and Chinese datasets. The development of TNFormer represents a notable advancement in text normalization task.