Documents
Poster
TOWARDS MULTI-DOMAIN FACE LANDMARK DETECTION WITH SYNTHETIC DATA FROM DIFFUSION MODEL
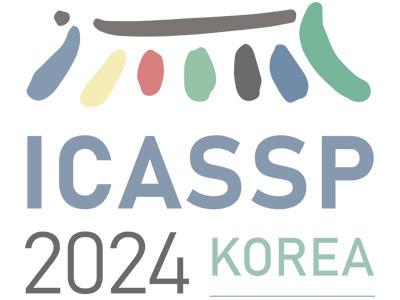
- Citation Author(s):
- Submitted by:
- YUANMING LI
- Last updated:
- 20 April 2024 - 4:40am
- Document Type:
- Poster
- Document Year:
- 2024
- Event:
- Presenters:
- YUANMING LI
- Paper Code:
- MLSP-P38.9
- Categories:
- Log in to post comments
Recently, deep learning-based facial landmark detection for in-the-wild faces has achieved significant improvement. However, there are still challenges in face landmark detection in other domains (\eg{} cartoon, caricature, etc). This is due to the scarcity of extensively annotated training data. To tackle this concern, we design a two-stage training approach that effectively leverages limited datasets and the pre-trained diffusion model to obtain aligned pairs of landmarks and face in multiple domains. In the first stage, we train a landmark-conditioned face generation model on a large dataset of real faces. In the second stage, we fine-tune the above model on a small dataset of image-landmark pairs with text prompts for controlling the domain. Our new designs enable our method to generate high-quality synthetic paired datasets from multiple domains while preserving the alignment between landmarks and facial features. Finally, we fine-tuned a pre-trained face landmark detection model on the synthetic dataset to achieve multi-domain face landmark detection. Our qualitative and quantitative results demonstrate that our method outperforms existing methods on multi-domain face landmark detection.