Documents
Poster
Training an Embedded Object Detector for Industrial Settings Without Real Images
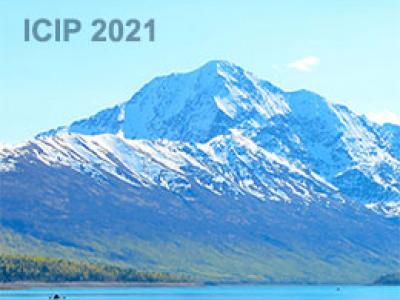
- Citation Author(s):
- Submitted by:
- Julia Cohen
- Last updated:
- 24 September 2021 - 11:19am
- Document Type:
- Poster
- Document Year:
- 2021
- Event:
- Presenters:
- Julia Cohen
- Categories:
- Log in to post comments
In an industrial environment, object detection is a challenging task due to the absence of real images and real-time requirements for the object detector, usually embedded in a mobile device. Using 3D models, it is however possible to create a synthetic dataset to train a neural network, although the performance on real images is limited by the domain gap. In this paper, we study the performance of a Convolutional Neural Network (CNN) designed to detect objects in real-time: Single-Shot Detector (SSD) with a MobileNet backbone. We train SSD with synthetic images only, and apply extensive data augmentation to reduce the domain gap between synthetic and real images. On the T-LESS dataset, SSD performs better than Mask R-CNN trained on the same synthetic images, with MobileNet-V2 and MobileNet-V3 Large as backbone. Our results also show the huge improvement enabled by an adequate augmentation strategy.