Documents
Poster
A UNIFIED DNN-BASED SYSTEM FOR INDUSTRIAL PIPELINE SEGMENTATION
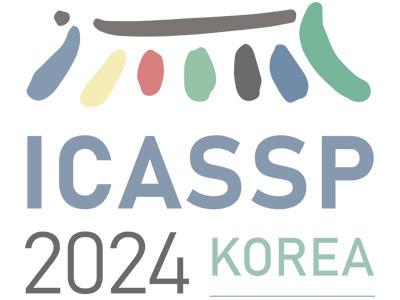
- Citation Author(s):
- Submitted by:
- Dimitrios Psarras
- Last updated:
- 14 April 2024 - 5:11pm
- Document Type:
- Poster
- Document Year:
- 2024
- Event:
- Presenters:
- Ioannis Pitas
- Paper Code:
- MLSP-P5.12
- Categories:
- Keywords:
- Log in to post comments
This paper presents a unified system tailored for autonomous pipe segmentation within an industrial setting. To this end, it is designed to analyze RGB images captured by Unmanned Aerial Vehicle (UAV)-mounted cameras to predict binary pipe segmentation maps. The overall proposed system consists of three main components: a) a Convolutional Neural Network (CNN) that is used to obtain initial estimates of the pipe segmentation maps, b) a point extraction module that acts on the outputs of the CNN to propose strong pipe class representatives in the input image space, and c) a foundation segmentation model, utilized to refine the initial estimations based on the proposed pipe class representatives. The architecture of the proposed system was specifically designed to ensure increased generalization ability in different, unknown environments, offering an effective solution to a well-known limitation of typical segmentation CNNs, at least in the pipe segmentation task. The effectiveness of the proposed system in this particular setting is evaluated by utilizing two pipe segmentation datasets, originating from two different industrial sites, which were manually annotated with the corresponding pipe segmentation maps. Experimental results demonstrate that the proposed system outperforms the baseline segmentation CNNs, demonstrating its remarkable generalization capabilities.