Documents
Presentation Slides
Video Enhancement Network Based on Max-pooling and Hierarchical Feature Fusion
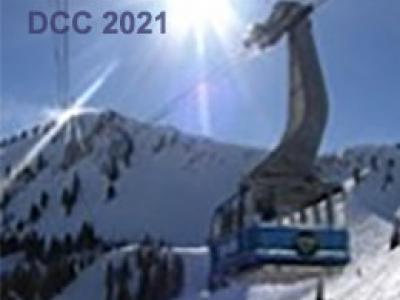
- Citation Author(s):
- Submitted by:
- Zheng Liu
- Last updated:
- 24 February 2021 - 9:11pm
- Document Type:
- Presentation Slides
- Document Year:
- 2021
- Event:
- Presenters:
- Zheng Liu
- Paper Code:
- 161
- Categories:
- Log in to post comments
In this paper, we propose an efficient convolution neural network to enhance the quality of video compressed by HEVC standard. The model is composed of a max-pooling module and a hierarchical feature fusion module. The max-pooling module extracts feature from different scales and enlarges the receptive field of the model without stacking too many convolution layers. And the hierarchical feature fusion module accurately aligns features from different scales and fuses them efficiently. Two modules are applied in the proposed network, our model reconstructs compressed video frames with higher visual quality. Besides, the model is constructed in the full convolution network, thus it can adapt to videos in variable resolutions. The experiment results show that the proposed model outperforms existing models in the terms of PSNR under the same dataset.
DCCPPT.pdf
