Documents
Poster
An Adapter-Based Unified Model for Multiple Spoken Language Processing Tasks
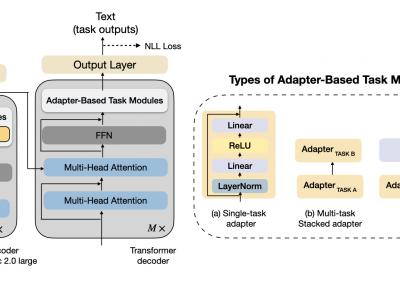
- Citation Author(s):
- Submitted by:
- Varsha Suresh
- Last updated:
- 4 April 2024 - 11:38pm
- Document Type:
- Poster
- Document Year:
- 2024
- Event:
- Presenters:
- Varsha Suresh
- Paper Code:
- 3826
- Categories:
- Log in to post comments
Self-supervised learning models have revolutionized the field of speech processing. However, the process of fine-tuning these models on downstream tasks requires substantial computational resources, particularly when dealing with multiple speech-processing tasks. In this paper, we explore the potential of adapter-based fine-tuning in developing a unified model capable of effectively handling multiple spoken language processing tasks. The tasks we investigate are Automatic Speech Recognition, Phoneme Recognition, Intent Classification, Slot Filling, and Spoken Emotion Recognition. We validate our approach through a series of experiments on the SUPERB benchmark, and our results indicate that adapter-based fine-tuning enables a single encoder-decoder model to perform multiple speech processing tasks with an average improvement of 18.4% across the five target tasks while staying efficient in terms of parameter updates.
suresh_3826.pdf
