Documents
Presentation Slides
DEEP MULTI-TASK LEARNING FOR GAIT-BASED BIOMETRICS
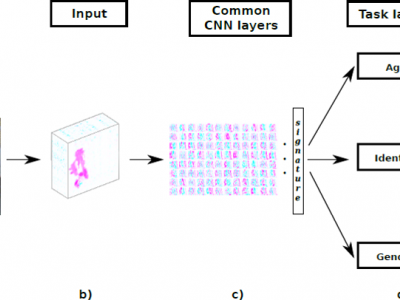
- Citation Author(s):
- Submitted by:
- Manuel Marin-Jimenez
- Last updated:
- 14 September 2017 - 9:42am
- Document Type:
- Presentation Slides
- Document Year:
- 2017
- Event:
- Presenters:
- Manuel J Marin-Jimenez
- Paper Code:
- 2011
- Categories:
- Log in to post comments
The task of identifying people by the way they walk is known as ‘gait recognition’. Although gait is mainly used for identification, additional tasks as gender recognition or age estimation may be addressed based on gait as well. In such cases, traditional approaches consider those tasks as independent ones, defining separated task-specific features and models for them. This paper shows that by training jointly more than one gait-based tasks, the identification task converges faster than when it is trained independently, and the recognition performance of multi-task models is equal or superior to more complex single-task ones. Our model is a multi-task CNN that receives as input a fixed-length sequence of optical flow channels and outputs several biometric features (identity, gender and age).