Documents
Poster
Exploiting spatial attention mechanism for improved depth completion and feature fusion in novel view synthesis
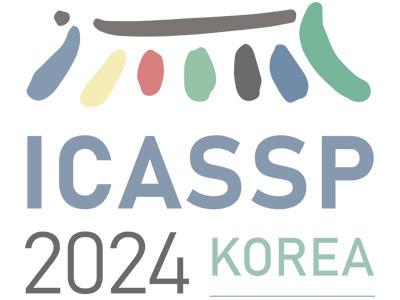
- Citation Author(s):
- Submitted by:
- MINH ANH TRUONG
- Last updated:
- 3 April 2024 - 12:34am
- Document Type:
- Poster
- Document Year:
- 2024
- Event:
- Presenters:
- TRUONG MINH ANH
- Paper Code:
- IVMSP-P20.7
- Categories:
- Log in to post comments
Many image-based rendering (IBR) methods rely on depth estimates obtained from structured light or time-of-flight depth sensors to synthesize novel views from sparse camera networks. However, these estimates often contain missing or noisy regions, resulting in an incorrect mapping between source and target views. This situation makes the fusion process more challenging, as the visual information is misaligned, inconsistent, or missing. In this work, we first implement a lightweight network based on the transformer, which is well-known for its capability to model long-range relationships within input data, to extract spatial features from color images. These features are then used to enhance the quality of completed depth maps. Furthermore, we combine a sequential deep neural network with a spatial attention mechanism to effectively fuse the projected features from multiple source viewpoints. This approach enables us to integrate information from an arbitrary number of source viewpoints as well as improve accuracy in synthesized views. Experimental results on challenging datasets demonstrate that our method achieves superior synthesized image quality compared to state-of-the-art (SOTA) methods. Github page: https://github.com/tmanh/nvs23.
Ban pdf.pdf
