Documents
Poster
EXPLORATION OF VISUAL PROMPT IN GROUNDED PRE-TRAINED OPEN-SET DETECTION
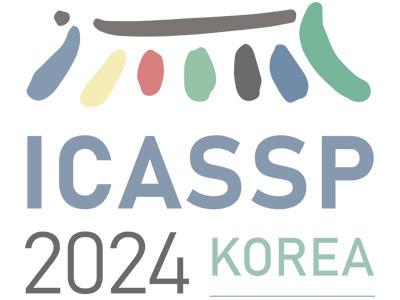
- DOI:
- 10.60864/1g54-pk82
- Citation Author(s):
- Submitted by:
- qibo chen
- Last updated:
- 3 April 2024 - 3:59am
- Document Type:
- Poster
- Document Year:
- 2024
- Event:
- Paper Code:
- MLSP-P9.4
- Categories:
- Log in to post comments
Text prompts are crucial for generalizing pre-trained open-set object detection models to new categories. However, current methods for text prompts are limited as they require manual feedback when generalizing to new categories, which restricts their ability to model complex scenes, often leading to incorrect detection results. To address this limitation, we propose a novel visual prompt method that learns new category knowledge from a few labeled images, which generalizes the pre-trained detection model to the new category. To allow visual prompts to represent new categories adequately, we propose a statistical-based prompt construction module that is not limited by predefined vocabulary lengths, thus allowing more vectors to be used when representing categories. We further utilize the category dictionaries in the pre-training dataset to design task-specific similarity dictionaries, which make visual prompts more discriminative. We evaluate the method on the ODinW dataset and show that it outperforms existing prompt learning methods and performs more consistently in combinatorial inference.