Documents
Poster
Linear Complexity Gibbs Sampling for Generalized Labeled Multi-Bernoulli Filtering
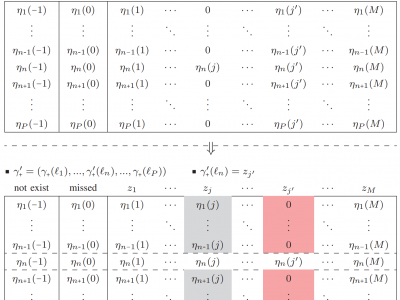
- DOI:
- 10.60864/9akq-x987
- Citation Author(s):
- Submitted by:
- Changbeom Shim
- Last updated:
- 16 April 2024 - 4:49pm
- Document Type:
- Poster
- Event:
- Presenters:
- Changbeom Shim
- Paper Code:
- SPTM-P8.2
- Categories:
- Log in to post comments
Generalized Labeled Multi-Bernoulli (GLMB) densities arise in a host of multi-object system applications analogous to Gaussians in single-object filtering. However, computing the GLMB filtering density requires solving NP-hard problems. To alleviate this computational bottleneck, we develop a linear complexity Gibbs sampling framework for GLMB density computation. Specifically, we propose a tempered Gibbs sampler that exploits the structure of the GLMB filtering density to achieve an O(T(P+M)) complexity, where T is the number of iterations of the algorithm, P and M are the number of hypothesized objects and measurements. This innovation enables the GLMB filter implementation to be reduced from an O(TP^2M) complexity to O(T(P+M+logT)+PM). Moreover, the proposed framework provides the flexibility for trade-offs between tracking performance and computational load. Convergence of the proposed Gibbs sampler is established, and numerical studies are presented to validate the proposed GLMB filter implementation.