Documents
Presentation Slides
MANIFOLD GRADIENT DESCENT SOLVES MULTI-CHANNEL SPARSE BLIND DECONVOLUTION PROVABLY AND EFFICIENTLY
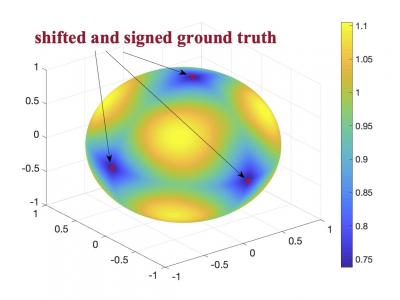
- Citation Author(s):
- Submitted by:
- Laixi Shi
- Last updated:
- 13 May 2020 - 5:30pm
- Document Type:
- Presentation Slides
- Document Year:
- 2020
- Event:
- Presenters:
- Laixi Shi
- Paper Code:
- 2240
- Categories:
- Keywords:
- Log in to post comments
Multi-channel sparse blind deconvolution refers to the problem of learning an unknown filter by observing its circulant convolutions with multiple input signals that are sparse. It is challenging to learn the filter efficiently due to the bilinear structure of the observations with respect to the unknown filter and inputs, leading to global ambiguities of identification. We propose a novel approach based on nonconvex optimization over the sphere manifold by minimizing a smooth surrogate of the sparsity-promoting loss function. It is demonstrated that manifold gradient descent with random initializations provably recovers the filter, up to scaling and shift ambiguities, as soon as the number of observations is sufficiently large under a suitable random data model. Numerical experiments are conducted to illustrate the efficiency of the proposed method with comparisons to existing methods.