Documents
Poster
Model-Free Learning of Optimal Beamformers for Passive IRS-Assisted Sumrate Maximization
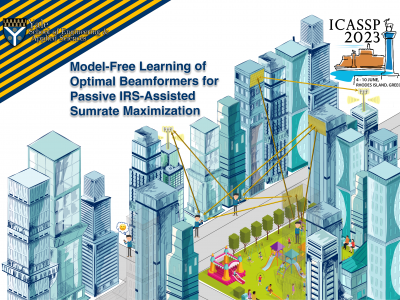
- Citation Author(s):
- Submitted by:
- Hassaan Hashmi
- Last updated:
- 31 May 2023 - 6:05pm
- Document Type:
- Poster
- Document Year:
- 2023
- Event:
- Presenters:
- Hassaan Hashmi
- Paper Code:
- SPCN-P3.11
- Categories:
- Keywords:
- Log in to post comments
Although Intelligent Reflective Surfaces (IRSs) are a cost-effective technology promising high spectral efficiency in future wireless networks, obtaining optimal IRS beamformers is a challenging problem with several practical limitations. Assuming fully-passive, sensing- free IRS operation, we introduce a new data-driven Zeroth-order Stochastic Gradient Ascent (ZoSGA) algorithm for sumrate optimization in an IRS-aided downlink setting. ZoSGA does not require access to channel model or network structure information, and enables learning of optimal long-term IRS beamformers jointly with standard short-term precoding, based only on conventional effective channel state information. Supported by state-of-the-art (SOTA) convergence analysis, detailed simulations confirm that ZoSGA exhibits SOTA empirical behavior as well, consistently outperforming standard fully model-based baselines, in a variety of scenarios.
ZoSGA_Poster.pdf
