Documents
Presentation Slides
NEAREST SUBSPACE SEARCH IN THE SIGNED CUMULATIVE DISTRIBUTION TRANSFORM SPACE FOR 1D SIGNAL CLASSIFICATION
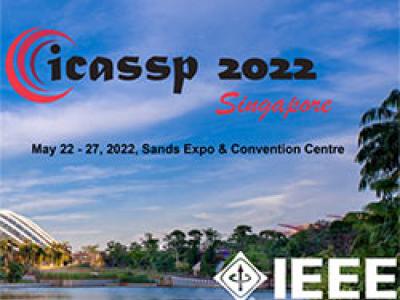
- Citation Author(s):
- Submitted by:
- Abu Hasnat Moha...
- Last updated:
- 5 May 2022 - 7:15pm
- Document Type:
- Presentation Slides
- Document Year:
- 2022
- Event:
- Presenters:
- Abu Hasnat Mohammad Rubaiyat
- Paper Code:
- MLSP-15.1
- Categories:
- Log in to post comments
This paper presents a new method to classify 1D signals using the signed cumulative distribution transform (SCDT). The proposed method exploits certain linearization properties of
the SCDT to render the problem easier to solve in the SCDT space. The method uses the nearest subspace search technique in the SCDT domain to provide a non-iterative, effective, and simple to implement classification algorithm. Experiments show that the proposed technique outperforms the state-of-the-art neural networks using a very low number of training samples and is also robust to out-of-distribution examples on simulated data. We also demonstrate the efficacy of the proposed technique in real-world applications by applying it to an ECG classification problem.