Documents
Poster
PRIORITIZING DATA ACQUISITION FOR END-TO-END SPEECH MODEL IMPROVEMENT
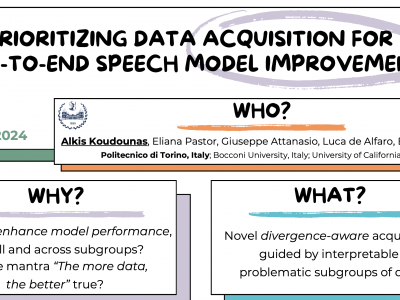
- Citation Author(s):
- Submitted by:
- Alkis Koudounas
- Last updated:
- 11 April 2024 - 10:11am
- Document Type:
- Poster
- Document Year:
- 2024
- Presenters:
- Alkis Koudounas
- Paper Code:
- MLSP-P1.8
- Categories:
- Log in to post comments
As speech processing moves toward more data-hungry models, data selection and acquisition become crucial to building better systems. Recent efforts have championed quantity over quality, following the mantra ``The more data, the better.''
However, not every data brings the same benefit. This paper proposes a data acquisition solution that yields better models with less data -- and lower cost.
Given a model, a task, and an objective to maximize, we propose a process with three steps. First, we assess the model's baseline performance on the task.
Second, we use efficient mining techniques to identify subgroups that maximize the target objective if acquired first as new samples. Being the subgroups interpretable, we can determine which samples to acquire. Third, we run incremental training sampling from those subgroups. Experiments with two state-of-the-art speech models for Intent Classification across two datasets in English and Italian show that our method is significantly better than random or complete acquisition and clustering-based techniques.