Documents
Supplementary material
PROGRESSIVE PASSIVE NON-LINE-OF-SIGHT IMAGING WITH LARGE MODEL PRIORS
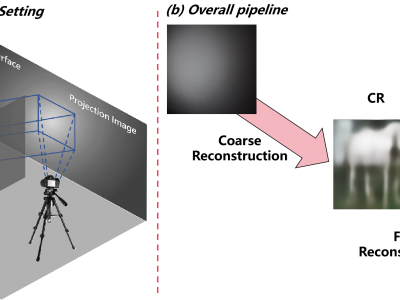
- DOI:
- 10.60864/rzq2-q916
- Citation Author(s):
- Submitted by:
- Xiaolong Du
- Last updated:
- 8 February 2024 - 6:10am
- Document Type:
- Supplementary material
- Document Year:
- 2024
- Presenters:
- Xiaolong Du
- Paper Code:
- ICIP-L1.0
- Categories:
- Keywords:
- Log in to post comments
Passive non-line-of-sight (NLOS) imaging has developed rapidly in recent years. However, existing models generally suffer from low-quality reconstruction due to the severe loss of information during the projection process. This paper proposes a two-stage passive NLOS imaging approach, aimed at reconstructing high-quality complicated hidden scenes. In the first stage, we train a coarse reconstruction network based on the optimal transport principle and using vector quantization to learn discrete priors for projection image encoding. This network generates a coarse reconstruction of the hidden image that seems blurry but contains the overall structure of the hidden image. In the second stage, we leverage a large, pre-trained text-to-image diffusion model to augment the coarse reconstruction and recover the image details. We elaborately design the controller modules and the loss functions of this fine reconstruction network to ensure the consistency between the generated image and the coarse reconstruction image. Comprehensive experiments on a large-scale passive NLOS dataset demonstrates the superiority of the proposed method.