Documents
Presentation Slides
Speaker Invariant Feature Extraction for Zero-Resource Languageswith Adversarial Learning
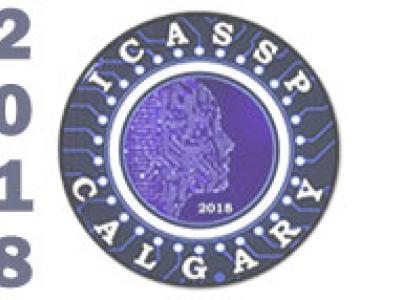
- Citation Author(s):
- Submitted by:
- Taira Tsuchiya
- Last updated:
- 13 April 2018 - 10:12am
- Document Type:
- Presentation Slides
- Document Year:
- 2018
- Event:
- Presenters:
- Taira Tsuchiya
- Paper Code:
- MLSP-L8.1
- Categories:
- Log in to post comments
We introduce a novel type of representation learning to obtain a speaker invariant feature for zero-resource languages. Speaker adaptation is an important technique to build a robust acoustic model. For a zero-resource language, however, conventional model-dependent speaker adaptation methods such as constrained maximum likelihood linear regression are insufficient because the acoustic model of the target language is not accessible. Therefore, we introduce a model-independent feature extraction based on a neural network. Specifically, we introduce a multi-task learning to a bottleneck feature-based approach to make bottleneck feature invariant to a change of speakers. The proposed network simultaneously tackles two tasks: phoneme and speaker classifications. This network trains a feature extractor in an adversarial manner to allow it to map input data into a discriminative representation to predict phonemes, whereas it is difficult to predict speakers. We conduct phone discriminant experiments in Zero Resource Speech Challenge 2017. Experimental results showed that our multi-task network yielded more discriminative features eliminating the variety in speakers.