Documents
Poster
UNCERTAINTY-GUIDED PERSON SEARCH MODEL WITH AUXILIARY SHALLOW FEATURE EXPLORATION
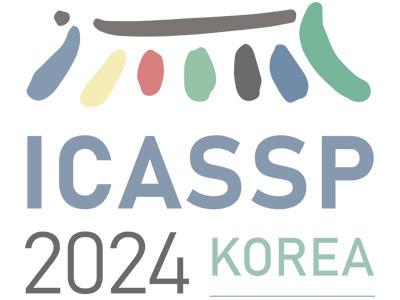
- Citation Author(s):
- Submitted by:
- Zongyi Li
- Last updated:
- 5 April 2024 - 7:10am
- Document Type:
- Poster
- Document Year:
- 2024
- Event:
- Presenters:
- Zongyi Li
- Categories:
- Log in to post comments
Person search is a unified system aimed at jointly localizing and identifying a person of interest from a gallery of whole scene images. Due to the inherent properties of the person search, it faces significant challenges of large-scale variations, inaccurate detection boxes, and crowded scenes. To address these issues, we proposed an uncertainty-guided framework coupled with auxiliary shallow feature exploration, which includes a shallow feature fusion module and an uncertaintyguided module. Firstly, considering the scales of the person are varied due to various scenes and their relative positions to the camera, a shallow feature fusion module is designed to extract multi-scale features to assist the re-id sub-task. Additionally, a self-distillation loss is proposed to align features across different scales. Furthermore, to alleviate the problem that the model can be easily affected by coarse samples resulting from crowded scenes and inaccurate detection boxes, we introduce an uncertainty guidance module to reduce the negative impact of these coarse targets. The experimental results demonstrate the effectiveness of our proposed methods on two benchmarks (i.e., CUHK-SYSU, and PRW).