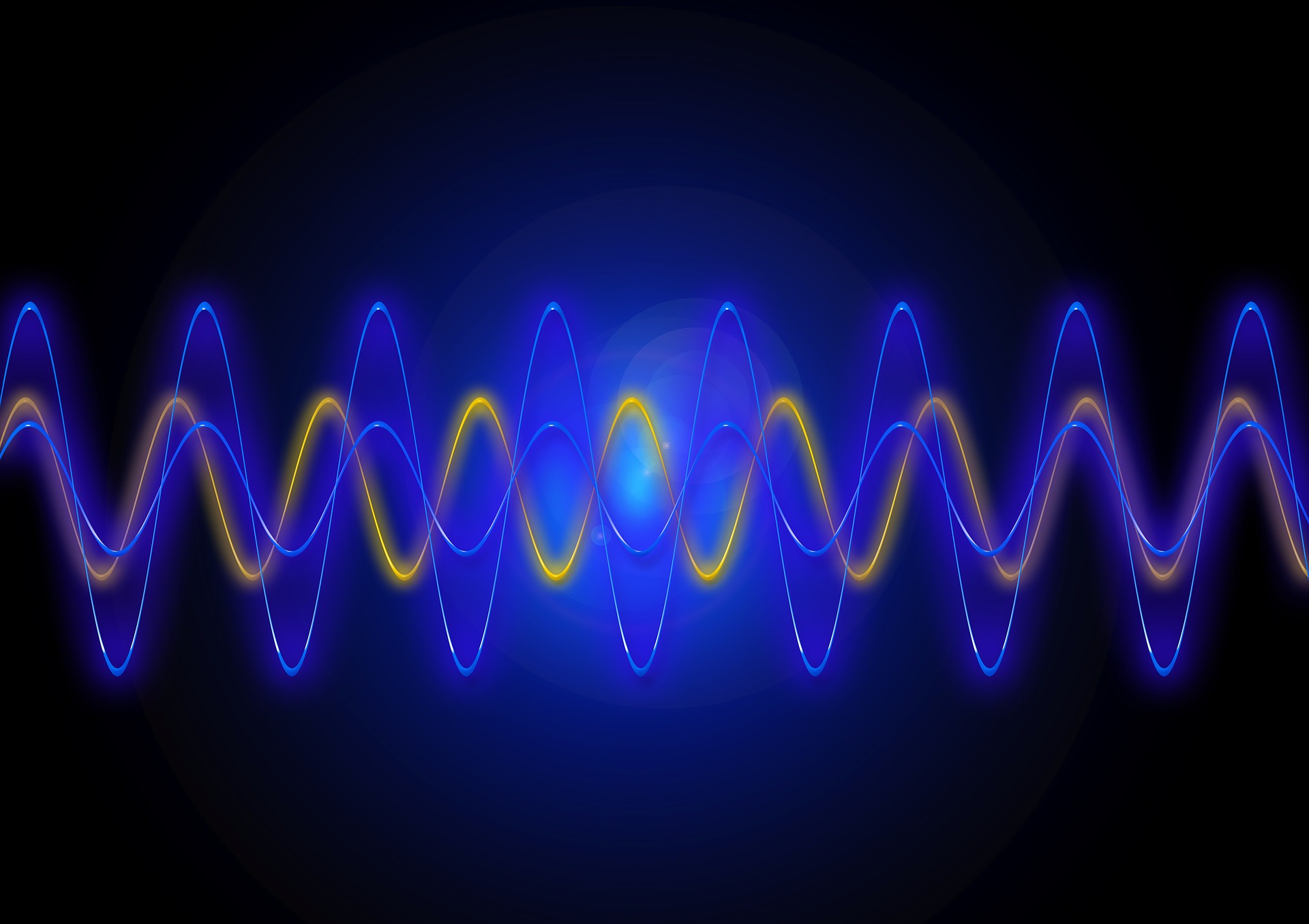
- Read more about VISUAL ADAPT FOR RGBD TRACKING
- Log in to post comments
Recent RGBD trackers have employed cueing techniques by overlaying Depth modality images as cues onto RGB modality images, which are then fed into the RGB-based model for tracking. However, the direct overlaying interaction method between modalities not only introduces more noise into the feature space but also exhibits the inadaptability of the RGB-based model to mixed-modality inputs. To address these issues, we introduce Visual Adapt for RGBD Tracking (VADT). Specifically, we maintain the input of the RGB-based model as the RGB modality.
- Categories:
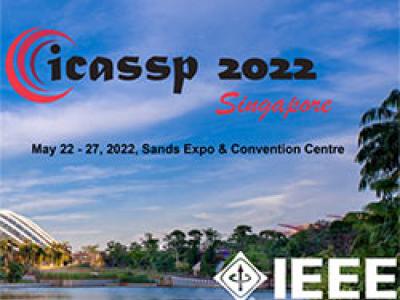
- Read more about SPARSE SUBSPACE TRACKING IN HIGH DIMENSIONS
- Log in to post comments
We studied the problem of sparse subspace tracking in the high-dimensional regime where the dimension is comparable to or much larger than the sample size. Leveraging power iteration and thresholding methods, a new provable algorithm called OPIT was derived for tracking the sparse principal subspace of data streams over time. We also presented a theoretical result on its convergence to verify its consistency in high dimensions. Several experiments were carried out on both synthetic and real data to demonstrate the effectiveness of OPIT.
- Categories:
- Read more about PARTICLE PHD FILTER BASED MULTI-TARGET TRACKING USING DISCRIMINATIVE GROUP-STRUCTURED DICTIONARY LEARNING
- Log in to post comments
Structured sparse representation has been recently found to achieve better efficiency and robustness in exploiting the target appearance model in tracking systems with both holistic and local information. Therefore, to better simultaneously discriminate multi-targets from their background, we propose a novel video-based multi-target tracking system that combines the particle probability hypothesis density (PHD) filter with discriminative group-structured dictionary learning.
- Categories: