- Transducers
- Spatial and Multichannel Audio
- Source Separation and Signal Enhancement
- Room Acoustics and Acoustic System Modeling
- Network Audio
- Audio for Multimedia
- Audio Processing Systems
- Audio Coding
- Audio Analysis and Synthesis
- Active Noise Control
- Auditory Modeling and Hearing Aids
- Bioacoustics and Medical Acoustics
- Music Signal Processing
- Loudspeaker and Microphone Array Signal Processing
- Echo Cancellation
- Content-Based Audio Processing
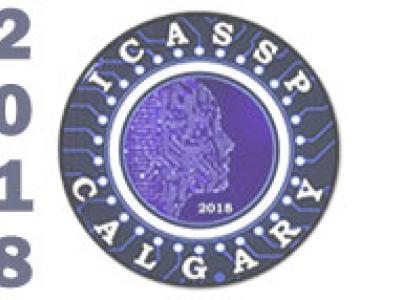
- Read more about REALIZING DIRECTIONAL SOUND SOURCE IN FDTD METHOD BY ESTIMATING INITIAL VALUE
- Log in to post comments
Wave-based acoustic simulation methods are studied actively for predicting acoustical phenomena. Finite-difference timedomain (FDTD) method is one of the most popular methods owing to its straightforwardness of calculating an impulse response. In an FDTD simulation, an omnidirectional sound source is usually adopted, which is not realistic because the real sound sources often have specific directivities. However, there is very little research on imposing a directional sound source into FDTD methods.
- Categories:
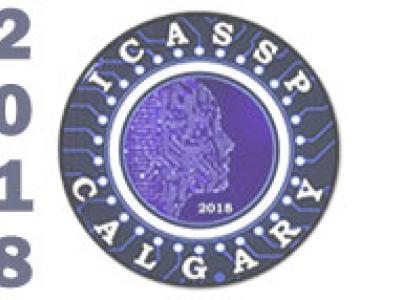
- Read more about Maximal Figure-of-Merit Embedding for Multi-label Audio Classification
- Log in to post comments
- Categories:
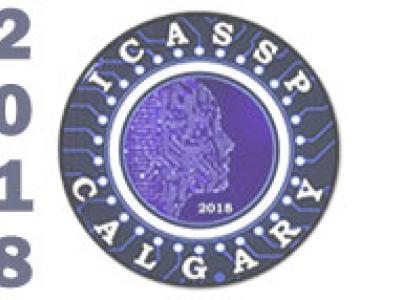
- Read more about Time-Frequency Networks for Audio Super-Resolution
- Log in to post comments
Audio super-resolution (a.k.a. bandwidth extension) is the challenging task of increasing the temporal resolution of audio signals. Recent deep networks approaches achieved promising results by modeling the task as a regression problem in either time or frequency domain. In this paper, we introduced Time-Frequency Network (TFNet), a deep network that utilizes supervision in both the time and frequency domain. We proposed a novel model architecture which allows the two domains to be jointly optimized.
- Categories:
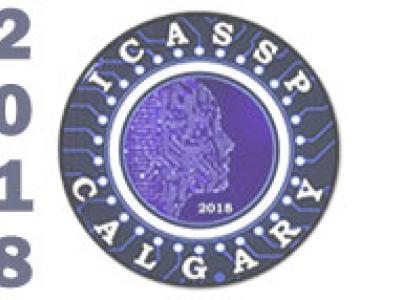
- Read more about Deep Clustering with Gated Convolutional Networks
- Log in to post comments
- Categories:
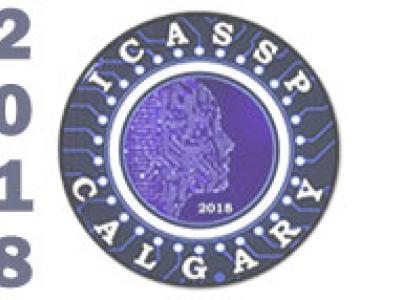
- Read more about EFFICIENT INTEGRATION OF FIXED BEAMFORMERS AND SPEECH SEPARATION NETWORKS FOR MULTICHANNEL FAR-FIELD SPEECH SEPARATION
- Log in to post comments
- Categories:
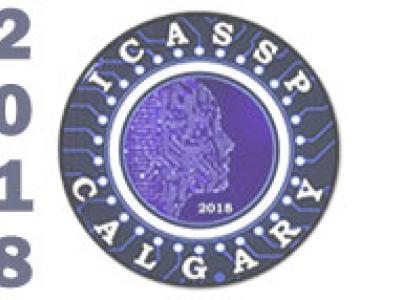
- Read more about AUDIO-VISUAL PERSON RECOGNITION IN MULTIMEDIA DATA FROM THE IARPA JANUS PROGRAM
- Log in to post comments
- Categories:
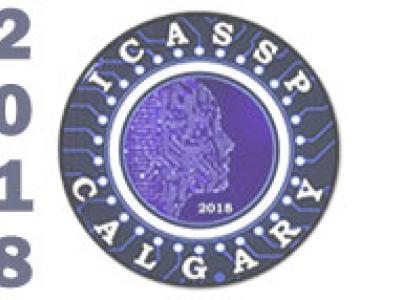
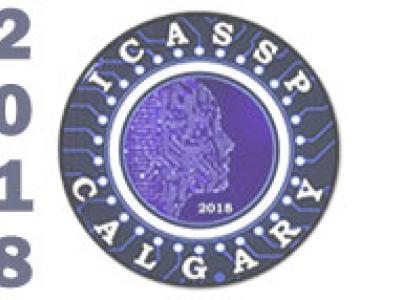
- Read more about A Novel Thresholding Technique for the Denoising of Multicomponent Signals
- Log in to post comments
This paper addresses the issues of the denoising and retrieval of the components of multicomponent signals from their short-time Fourier transform (STFT). After having recalled the hard-thresholding technique, in the STFT context, we develop a new thresholding technique by exploiting some limitations of the former. Numerical experiments illustrating the benefits of the proposed method to retrieve the modes of noisy multicomponent signals conclude the paper.
- Categories:
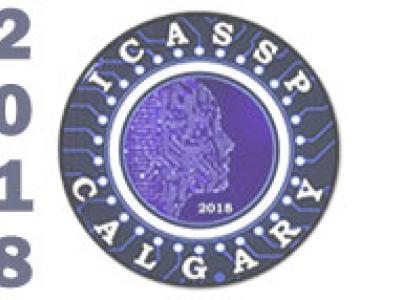
- Read more about Benchmarking Uncertainty Estimates with Deep Reinforcement Learning for Dialogue Policy Optimisation
- Log in to post comments
- Categories:
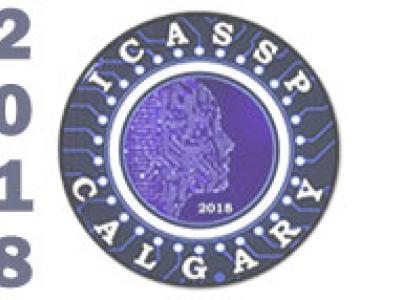
- Read more about SCALABLE SENTIMENT FOR SEQUENCE-TO-SEQUENCE CHATBOT RESPONSE WITH PERFORMANCE ANALYSIS
- Log in to post comments
Conventional seq2seq chatbot models only try to find the sentences with the highest probabilities conditioned on the input sequences, without considering the sentiment of the output sentences. Some research works trying to modify the sentiment of the output sequences were reported. In this paper, we propose five models to scale or adjust the sentiment of the chatbot response: persona-based model, reinforcement learning, plug and play model, sentiment transformation network and cycleGAN, all based on the conventional seq2seq model.
- Categories: