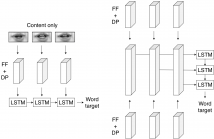
We present a novel lipreading system that improves on the task of speaker-independent word recognition by decoupling motion and content dynamics. We achieve this by implementing a deep learning architecture that uses two distinct pipelines to process motion and content and subsequently merges them, implementing an end-to-end trainable system that performs fusion of independently learned representations. We obtain a average relative word accuracy improvement of ≈6.8% on unseen speakers and of ≈3.3% on known speakers, with respect to a baseline which uses a standard architecture.
- Categories:
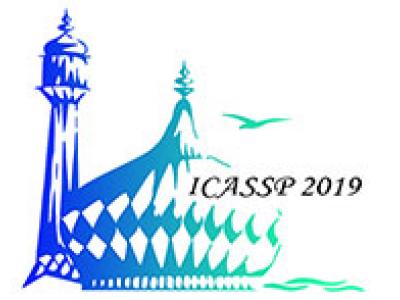
- Read more about Knowledge Distillation Using Output Errors for Self-Attention ASR Models
- Log in to post comments
Most automatic speech recognition (ASR) neural network models are not suitable for mobile devices due to their large model sizes. Therefore, it is required to reduce the model size to meet the limited hardware resources. In this study, we investigate sequence-level knowledge distillation techniques of self-attention ASR models for model compression.
- Categories:
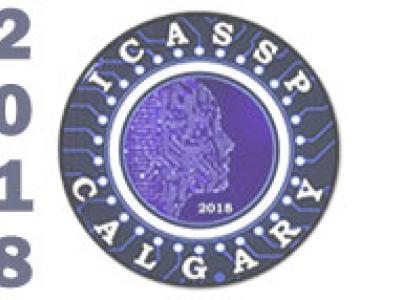
- Read more about RECOGNIZING ZERO-RESOURCED LANGUAGES BASED ON MISMATCHED MACHINE TRANSCRIPTIONS
- Log in to post comments
Mismatched crowdsourcing based probabilistic human transcription has been proposed recently for training and adapting acoustic models for zero-resourced languages where we do not have any native transcriptions. This paper describes a machine transcription based phone recognition system for recognizing zero-resourced languages and compares it with baseline systems of MAP adaptation and semi-supervised self training.
Poster.pdf

- Categories:
Deep learning has significantly advanced state-of-the-art of speech
recognition in the past few years. However, compared to conventional
Gaussian mixture acoustic models, neural network models are
usually much larger, and are therefore not very deployable in embedded
devices. Previously, we investigated a compact highway deep
neural network (HDNN) for acoustic modelling, which is a type
of depth-gated feedforward neural network. We have shown that
HDNN-based acoustic models can achieve comparable recognition
- Categories: