Documents
Poster
A Dynamic Latent Variable Model for Source Separation
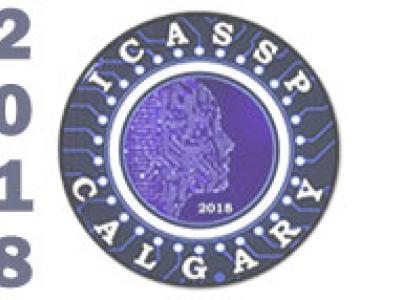
- Citation Author(s):
- Submitted by:
- Anurendra Kumar
- Last updated:
- 19 April 2018 - 2:17pm
- Document Type:
- Poster
- Document Year:
- 2018
- Event:
- Presenters:
- Pavan Karjol
- Paper Code:
- 2166
- Categories:
- Log in to post comments
We propose a novel latent variable model for learning latent bases for time-varying non-negative data. Our model uses a mixture multinomial as the likelihood function and proposes a Dirichlet distribution with dynamic parameters as a prior, which we call the dynamic Dirichlet prior. An expectation maximization (EM) algorithm is developed for estimating the parameters of the proposed model. Furthermore, we connect our proposed dynamic Dirichlet latent variable model (dynamic DLVM) to the two popular latent basis learning methods - probabilistic latent component analysis (PLCA) and non-negative matrix factorization (NMF). We show that (i) PLCA is a special case of the dynamic DLVM, and (ii) dynamic DLVM can be interpreted as a dynamic version of NMF. The effectiveness of the proposed model is demonstrated through extensive experiments on speaker source separation, and speech-noise separation. In both cases, our method performs better than relevant and competitive baselines. For speaker separation, dynamic DLVM shows 1:38 dB improvement in terms of source to interference ratio, and 1 dB improvement in source to artifact ratio
ICASSP_18.pdf
