Documents
Research Manuscript
Effectiveness of random deep feature selection for securing image manipulation detectors against adversarial examples
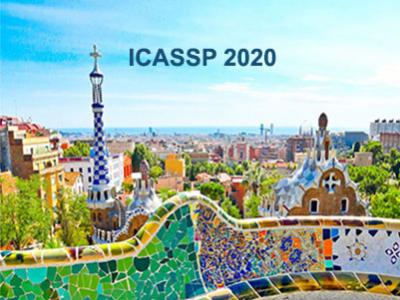
- Citation Author(s):
- Submitted by:
- Ehsan Nowroozi
- Last updated:
- 30 January 2020 - 12:06pm
- Document Type:
- Research Manuscript
- Document Year:
- 2020
- Event:
- Presenters:
- Mauro Barni, Ehsan Nowroozi, Benedetta Tondi, Bowen Zhang
- Paper Code:
- 3729
- Categories:
- Log in to post comments
We investigate if the random feature selection approach proposed in [1] to improve the robustness of forensic detectors to targeted attacks, can be extended to detectors based on deep learning features. In particular, we study the transferability of adversarial examples targeting an original CNN image manipulation detector to other detectors (a fully connected neural network and a linear SVM) that rely on a random subset of the features extracted from the flatten layer of the original network. The results we got by considering three image manipulation detection tasks (resizing, median filtering and adaptive histogram equalization), two original network architectures and three classes of attacks, show that feature randomization helps to hinder attack transferability, even if, in some cases, simply changing the architecture of the detector, or even retraining the detector is enough to prevent the transferability of the attacks.