Documents
Poster
AN END-TO-END APPROACH TO JOINT SOCIAL SIGNAL DETECTION AND AUTOMATIC SPEECH RECOGNITION
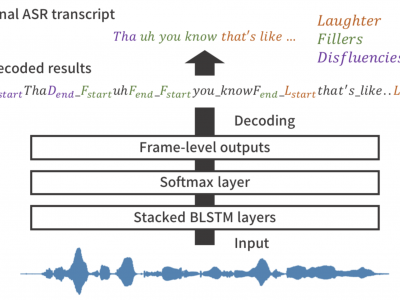
- Citation Author(s):
- Submitted by:
- Hirofumi Inaguma
- Last updated:
- 17 April 2018 - 7:49pm
- Document Type:
- Poster
- Document Year:
- 2018
- Event:
- Presenters:
- Hirofumi Inaguma
- Paper Code:
- HLT-P3.8
- Categories:
- Log in to post comments
Social signals such as laughter and fillers are often observed in natural conversation, and they play various roles in human-to-human communication. Detecting these events is useful for transcription systems to generate rich transcription and for dialogue systems to behave as we do such as synchronized laughing or attentive listening. We have studied an end-to-end approach to directly detect social signals from speech by using connectionist temporal classification (CTC), which is one of the end-to-end sequence labelling models. In this work, we propose a unified framework that integrates social signal detection (SSD) and automatic speech recognition (ASR). We investigate several reference labelling methods regarding social signals. Experimental evaluations demonstrate that our end-to-end framework significantly outperforms the conventional DNN-HMM system with regard to SSD performance as well as the character error rate (CER).