Documents
Poster
Poster
Improving Semi-Supervised Classification for Low-Resource Speech Interaction Applications
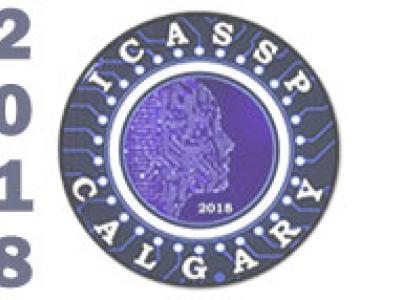
- Citation Author(s):
- Submitted by:
- Manoj Kumar
- Last updated:
- 12 April 2018 - 6:08pm
- Document Type:
- Poster
- Document Year:
- 2018
- Event:
- Presenters:
- Manoj Kumar
- Categories:
- Log in to post comments
We propose a semi-supervised learning method to improve classification performance in scenarios with limited labeled
data. We employ adaptation strategies such as entropy-filtering and self-training, and show that our method achieves
up to 17.2% relative improvement in UAR for a multi-class problem. We apply our method to two different tasks: speaker clustering for adult-child interactions during autism assessment sessions, and a variation of the language identification task (LID). We show that in both tasks our method improves classification accuracy while using lesser training data than the baseline and demonstrate the robustness of our setup to the degree of adaptation by controlling the threshold on uncertainty of classification.