Documents
Poster
SAMPLERNN-BASED NEURAL VOCODER FOR STATISTICAL PARAMETRIC SPEECH SYNTHESIS
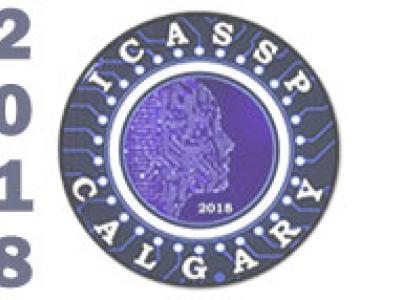
- Citation Author(s):
- Submitted by:
- Yang Ai
- Last updated:
- 13 April 2018 - 3:29am
- Document Type:
- Poster
- Document Year:
- 2018
- Event:
- Presenters:
- Yang Ai
- Paper Code:
- 1671
- Categories:
- Log in to post comments
This paper presents a SampleRNN-based neural vocoder for statistical parametric speech synthesis. This method utilizes a conditional SampleRNN model composed of a hierarchical structure of GRU layers and feed-forward layers to capture long-span dependencies between acoustic features and waveform sequences. Compared with conventional vocoders based on the source-filter model, our proposed vocoder is trained without assumptions derived from the prior knowledge of speech production and is able to provide a better modeling and recovery of phase information. Objective and subjective evaluations are conducted on two corpora. Experimental results suggested that our proposed vocoder can achieve higher quality of synthetic speech than the STRAIGHT vocoder and a WaveNet-based neural vocoder with similar run-time efficiency, no matter natural or predicted acoustic features are used as inputs.